Thousands of human sequences provide deep insight into single genomes
A massive genome-sequencing and analysis effort has produced the most comprehensive sets of data and tools for understanding human genetic variation so far. The resource will be invaluable to biologists of every stripe.
by Deanna M. ChurchWhat do the differences between each person’s genetic code mean for their individual development and health? Several factors have hampered researchers’ ability to answer this question. First, understanding genetic variation requires analysing huge numbers of sequences, because we carry many rare variants [1]. Most of these have no effect, with just a few causing genetic diseases. Second, most of our understanding of genetic variation has come from studying single nucleotide variants (SNVs), but structural variants — more than 50 nucleotides long — can have a larger impact on physiological traits, and are major contributors to disease [2]. Third, we lack an understanding of variation outside protein-coding sequences. In four papers in Nature, the genome aggregation database (gnomAD) consortium [3] [–] [6] sets out to address these gaps in knowledge.
The gnomAD project is the successor to the game-changing exome aggregation consortium (ExAC) [7] project, which catalogued genetic variation in the protein-coding parts of the genome, called exomes, from more than 60,000 people (Fig. 1). ExAC set a new standard for harmonized analysis — bringing in data from diverse projects for reanalysis in a common pipeline — and for data sharing. The ExAC data were available to scientists well before the project’s publication in 2016, and it has had a profound impact on how researchers, physicians and genetic counsellors interpret the genomes of people with genetic diseases.
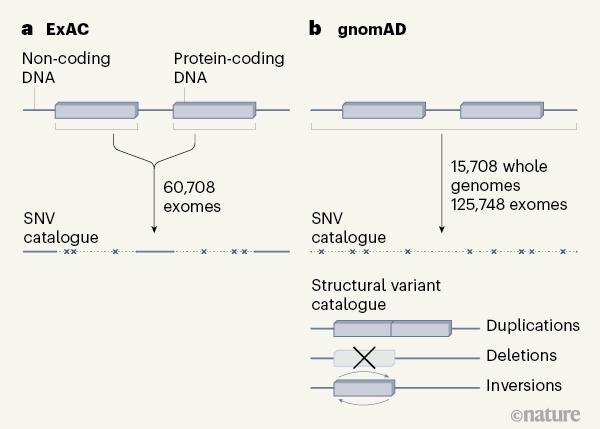
In the first of the current papers, Karczewski et al. [3] describe the gnomAD consortium’s collection of 125,748 exomes and 15,708 whole genomes. The move to sequencing whole genomes is especially exciting, because analysis of non-coding sequences provides information about both structural variation and variation in DNA sequences that regulate gene expression — described in the companion papers. The gnomAD resource includes sequences from diverse populations, including individuals from Asia and Africa. However, as the authors note, representation from more diverse populations is still needed to obtain the full spectrum of human variation and to capture more population-specific variation.
Karczewski et al. went on to analyse the protein-coding variants in their data set. They built on a metric developed by the ExAC group to assess whether a gene can ‘tolerate’ variants that are predicted to prevent the normal functions of the protein it encodes — that is, whether these predicted loss-of-function (pLoF) variants have little to no effect on physiology, or cause serious health issues or death. This type of analysis is useful because genes that are intolerant to LoF might be essential for life, or their mutation could cause genetic diseases.
The ExAC metric measures how many pLoF variants are observed in a gene across a population, compared with how many are expected given the rate at which mutations arise in genomes throughout evolution. However, because pLoF variants are so rare, 60,000 exomes was not enough to definitively say whether all the genes studied — particularly small genes — are intolerant to pLoF. The data were therefore expressed as the probability that a given gene would tolerate pLoF.
By contrast, the increased cohort size in gnomAD allows for a more-direct measure of gene tolerance to LoF. Karczewski et al. binned genes into ten groups according to the frequency of pLoF variants they contained compared with those expected, producing a spectrum of pLoF tolerance. The larger sample size means that gene length is less of a problem in the gnomAD analysis, but even so, the authors could not definitively assess pLoF frequency in the 30% of genes that were expected to have few pLoF variants.
Despite this limitation, the group use their approach to gain fresh insights into the genetics of disease. For example, they found rare variants in genes that do not tolerate LoF more often in people who have an intellectual disability or autism spectrum disorder than in people who do not. These data might help researchers to understand the complex genetic structure that underlies these traits.
In the second paper of the collection, Cummings et al. [4] investigated why genes that seem intolerant to pLoF can sometimes carry these variants with apparently little consequence. Genes can be transcribed in different ways, with some protein-coding regions (exons) expressed only in a limited fashion. Cummings and colleagues demonstrated that, when an individual carries a pLoF variant in an ‘intolerant’ gene, the variant is often in an exon that shows this restricted expression, thus limiting its effect.
In the third paper, Minikel et al. [5] assessed how the pLoF database might improve our ability to identify genetic targets for drugs. The identification of individuals who carry two pLoF variants in a given gene is desirable in drug discovery — if these individuals also exhibit a change in a particular trait, it provides evidence that the gene could be a good drug target [8]. The group showed that there are still many errors when identifying pLoF variants; that quality control is needed when identifying these variants; and that instances of an individual carrying two pLoF variants in the same gene are sufficiently rare that we will need cohorts roughly 1,000 times bigger than gnomAD to gather definitive evidence of their existence in most genes.
One of the most exciting aspects of the gnomAD project is the production of a catalogue of structural variants, described in the final paper by Collins and co-workers [6]. There have been excellent efforts at cataloguing structural variants using long-read sequencing technology [9]. However, sample sizes have been small, owing to the expense and lack of standardized analysis pipelines for this approach — although I expect this situation to improve in the near future. By contrast, identifying structural variants in short-read sequences is technically challenging, because the variants are often larger than a typical short sequence read, and they can arise through a variety of mutational mechanisms, resulting in many variant types (duplication, deletion or inversion of DNA, for instance) that each leave different footprints in the genome. This has led to the development of many tools for identifying structural variants from short reads, but no ‘standard’ pipeline.
Collins et al. sought to remedy this problem by creating a pipeline that allows for harmonized analysis over thousands of genomes; this could become the industry standard for structural-variant detection from short-read sequences on a population scale. The authors generated a catalogue of more than 300,000 high-quality structural variants — more than twice as many as previous analyses. They then began to assess the contribution of structural variants to physiological traits. This analysis revealed some evidence for natural selection against structural variants in non-coding sequences that control gene expression. Unsurprisingly, selection against structural variants was stronger in protein-coding regions. This suggests that more variation is tolerated in non-coding than in coding regions, and that even-larger cohorts (or other approaches) will be needed to begin to robustly dissect non-coding variation. The authors also found that structural variants account for roughly one-quarter of protein-truncating events.
The routine analysis of structural variants, integrated with analysis of SNVs and gene expression, will be crucial for interpreting individual genomes. Collins et al. have taken an important step in this direction, and the gnomAD resource provides tools for others to continue on this path.
An interesting, recurring theme in these papers is that — despite the size of the cohort — we still lack the numbers required for many analyses. The sequencing of ever-larger cohorts should no doubt continue. However, this approach alone will not enable us to fully understand the relationships between human genetics and traits at both cellular and organismal levels. We need scalable approaches to program genetic variation into human cells, and well-characterized cellular traits that can be monitored to allow us to directly interrogate the physiological impact of this variation. Such interventional biology will substantially augment population genetics and accelerate our understanding of human biology.
The gnomAD consortium has already made its data publicly available. The impact that the project will have on science goes well beyond the current collection, which includes not only the papers in this issue, but several published in Nature’s sister journals (go.nature.com/2zgfxr2). The gnomAD resource, like ExAC before it, will change how we interpret individual genomes. The consortium’s work has revealed how much information about human variation we had been missing and has provided tools that help us to better understand the genome at both the population and individual level. I can’t wait to see what comes next.
Nature 581, 385-386 (2020)
doi: 10.1038/d41586-020-01485-4
References
1. Auton, A. et al. Nature 526, 68–74 (2015).
2. Chiang, C. et al. Nature Genet. 49, 692–699 (2017).
3. Karczewski, K. J. et al. Nature 581, 434–443 (2020).
4. Cummings, B. B. et al. Nature 581, 452–458 (2020).
5. Minikel, E. V. et al. Nature 581, 459–464 (2020).
6. Collins, R. L. et al. Nature 581, 444–451 (2020).
7. Lek, M. et al. Nature 536, 285–291 (2016).
8. Plenge, R. M., Scolnick, E. M. & Altshuler, D. Nature Rev. Drug Discov. 12, 581–594 (2013).
9. Chaisson, M. J. P. et al. Nature Commun. 10, 1784 (2019).
Competing Financial Interests
D.M.C. is employed by and a shareholder in Inscritpa, Inc.