Scientific Pressure and the Yale COVID Poop Study
Flaws revealed in another new COVID study from an elite university
by Alexander Danvers Ph.D.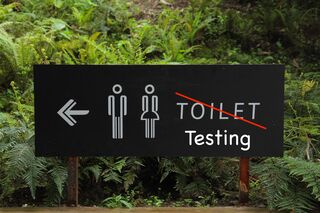
When a person with COVID-19 poops, some of the virus’ genetic material can be detected through chemical testing. You can also detect COVID-19 RNA in an area’s sewage. This led Yale researchers to come up with a clever idea: what if you could predict outbreaks of COVID-19 from analyzing an area’s sewage? As Yale researcher Jordan Peccia and colleagues report in a new preprint, it does! And not only does it work, but poop analysis *nearly perfectly* predicts outbreaks three days later!
This finding is already starting to make the rounds of the hype train, with a Fox News headline and nearly 2 million views of the study on twitter (according to a researcher who tweeted out a link to the study). But—and stop me if you see a theme here—the statistics were incorrect and misleading, hugely overestimating the ability of poop analysis to predict COVID-19 outbreaks. As in several recent studies out of Stanford, the need to make headlines seemed to trump the need for caution in reporting results.
First, a brief, not-too-technical explanation of the issue: the authors report that the amount of COVID-19 RNA in poop predicts the number of cases in an area with an R2 = .99. This statistic represents how much of the variability in an outcome (in this case, number of COVID-19 cases) can be predicted by other variables (in this case, amount of RNA in poop). The scale is 0-1, meaning that you can roughly interpret the value reported by the Yale researchers as saying their prediction is 99% accurate.
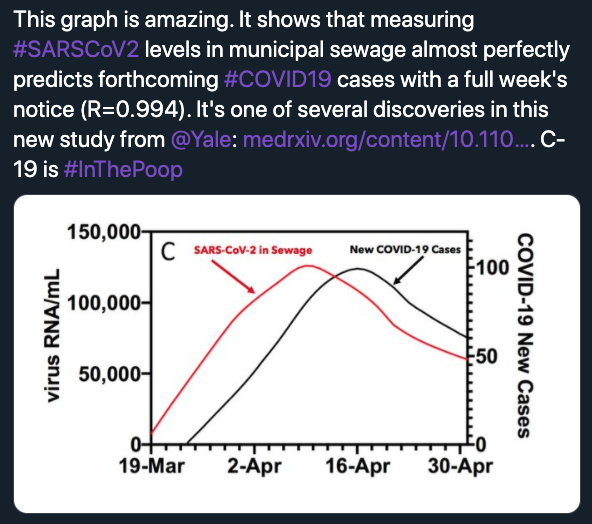
That’s insanely high. In psychology, we’d consider an R2 = .10 to be high. That’s about the relationship you get between someone saying they’re extraverted and how much time they spend talking in their daily life. The Yale researchers reported “too good to be true” results. And, like most “too good to be true” results, they weren’t true.
The problem was that, instead of calculating the correlation using the actual data points they collected, the Yale researchers first smoothed the data—which removes variability and makes it much easier to predict. Instead of analyzing their raw data—the actual measurements they made, without any manipulation—they decided to analyze data that removed most of the unpredictable parts of their measurement. This is a misleading and inappropriate approach.
You can see this in the screenshots I've got here: the one with just the curves makes it look like all the data lines up nearly perfectly. But that's not the actual measurements. The actual measurements are the dots in the screenshot below--and they don't line up perfectly on the curves at all. What should have been analyzed is the measurements (the dots), not the curves.
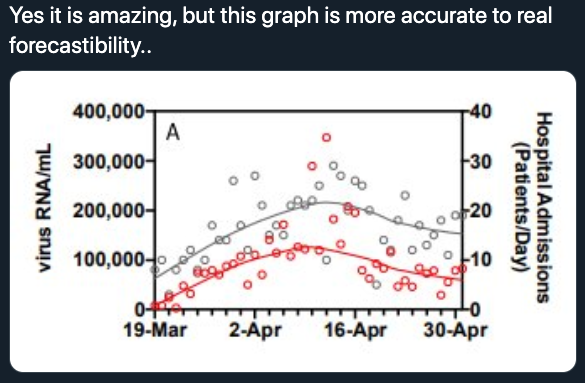
This was immediately pointed out by other researchers on twitter. A small group even tried to reverse engineer the data (through automatic extraction of data from the graph in the paper) and calculate what the appropriate result would be. Their conclusion: somewhere in the neighborhood of 15-40% of the variance in COVID-19 cases explained. In other words, the accuracy reported was off by potentially 84%.
Why do we see Yale researcher, like recent work by Stanford researchers, using sloppy statistics to make over-hyped claims? In part, it’s because of what it takes to become a Yale or Stanford researcher. Being a research professor at an elite U.S. university means continually churning out ground-breaking research findings. Researchers at elite universities work under the Paradigm of Routine Discovery: their colleagues, university officials, granting bodies, and even they themselves seem to expect that big scientific findings can be reliably generated over and over by the same small group of elite researchers. The implicit idea is that a Big Name researcher at a Big Name university can figure out a system or program to routinely push the outer bounds of human knowledge—and if these researchers can’t, the university can always hire another set of researchers who can.
Yet expecting researchers to deliver ground-breaking new effects every year is out of line with the pace of insight and progress in science. In his book Representing and Intervening, Philosopher of Science Ian Hacking gives a ballpark estimation that all of progress in physics (from Newton up to 1983, when the book was published) was based on 40 effects. Coming out with even one of these in an entire scientific career, therefore, would be a huge accomplishment—never mind one a year (or more) until you’re up for tenure.
My concern is that, when this kind of productivity pressure is placed on researchers, sloppiness becomes inevitable. I believe that you can have poorly done science without having a lot of “bad” scientists. Given the wrong incentive system, you can blindly select for research that is poorly done. In that environment, each level of promotion in the academic chain is potentially weeding out more people who work slowly but carefully—and this effect may be accentuated at elite universities.
The key takeaway here isn’t that the Yale researchers who conducted the poop study are bad. It’s very thoughtful and creative, and even being able to predict COVID-19 outbreaks with 15% accuracy is useful! The takeaway is that the behavior of scientists is governed by incentives, and these need to be better aligned with the production of high-quality science. Until they are, people need to bear these incentives in mind when they read about new scientific findings.