Using big data to combat catastrophes
by Science X staff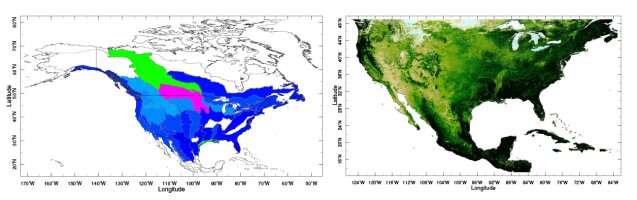
In March 1989, a tripped circuit in the Hydro-Québec power grid left 6 million people without electricity. A week earlier, an unusually harsh snowstorm had strained the region; the day before, a solar flare and accompanying release of plasma and magnetic field sent a mountain of energy propelling toward Earth at a million miles an hour.
The complex interactions of these interconnected systems—environmental science, space weather and solar activity—pushed the electric power grid to a tipping point that could not be understood within any single one of those systems.
The Predictive Risk Investigation System for Multilayer Dynamic Interconnection Analysis (PRISM), funded by the National Science Foundation, aims to harness data in order to identify risk factors across domains for catastrophic events such as the 1989 blackout, which impacted transportation, food, water, health and finance and racked up costs exceeding $2 billion.
Columbia University's International Research Institute for Climate and Society, part of the Earth Institute, is one of the ten collaborating institutions on the project.
The PRISM team—comprising experts from data science, statistics, computer science, finance, energy, agriculture, ecology, hydrology, climate and space weather—will integrate large data sets across different sectors to improve risk prediction. Such an undertaking requires significant computing and data curation capabilities, something extremely well suited to the IRI's Data Library platform.
"This project brings in datasets from very diverse domains of science that use different ways to describe time and space and that use different file formats," says IRI's Rémi Cousin. "Our Data Library removes such complexities by placing all data in an interoperable framework—one which projects scientists are able to query online to feed into their own analytical programs."
Once the data is incorporated into the Data Library, Cousin and his collaborators will use cutting-edge analysis to identify what they've called critical risk indicators—quantifiable information associated with risk exposure, particularly for potential catastrophes. They'll also employ machine learning to look for anomalies in the data that might lead to new insights.
"We want to focus our attention on these worst-case scenarios and the risks associated with them, and how we might measure their likelihood," said Cornell University's David S. Matteson, who is a principal investigator on the two-year, $2.4 million project.
"Our hope is that by identifying systemically important critical risks—those that tie together different domains and have the biggest spillover potential—we will have the most widespread impact in terms of controlling those risks," Matteson said.
If systems had been in place to recognize the heightened risks caused by the snowstorm and the solar flare, the 1989 power outage may have been averted or at least minimized. Similarly, understanding the ways it affected systems such as health care and transportation could help policy makers plan a more effective response.
The multidisciplinary approach is essential because today's world is composed of highly interconnected and interdependent systems, and no single expert is equipped to identify the signs of risk or the full impact of catastrophes.
The researchers will then focus their efforts on identifying risk interconnections, and systemically important risk indicators across the different domains, in order to both predict potential hazards and to lessen the possible system-wide losses once they've occurred. They plan to examine known risk indicators and apply data science to identify new ones.
"Our goal is ultimately to help create early warning systems for catastrophes and improve preparedness for these devastating events," Cousin said. "We plan to integrate the results of the project and make them publicly accessible via the Data Library."
Provided by Earth Institute, Columbia University
This story is republished courtesy of Earth Institute, Columbia University http://blogs.ei.columbia.edu.