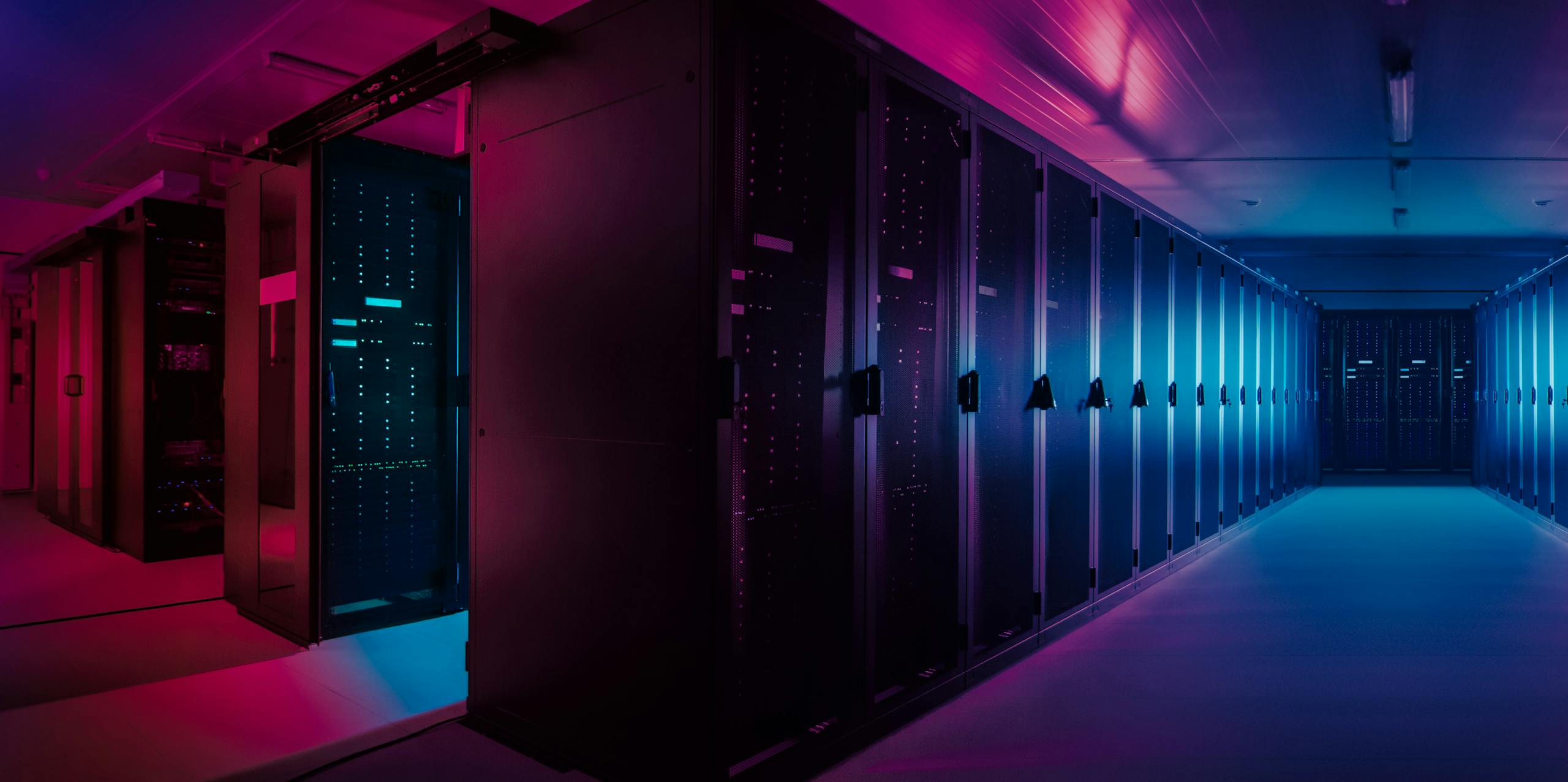
AI algorithms intended to root out welfare fraud often end up punishing the poor instead
by Michele GilmanPresident Donald Trump recently suggested there is “tremendous fraud” in government welfare programs.
Although there’s very little evidence to back up his claim, he’s hardly the first politician – conservative or liberal – to vow to crack down on fraud and waste in America’s social safety net.
States – which are charged with distributing and overseeing many federally funded benefits – are taking these fraud accusations seriously. They are increasingly turning to artificial intelligence and other automated systems to determine benefits eligibility and ferret out fraud in a variety of benefits programs, from food stamps and Medicaid to unemployment insurance.
Of course, government agencies should ensure that taxpayer dollars are spent effectively. The problem is these automated decision-making systems are sometimes rife with errors and designed in ways that punish the poor for being poor, leading to tragic results.
As a clinical law professor who has researched safety net programs and has represented low-income clients in public benefits cases for over 20 years, I believe it’s essential these systems are designed in ways that are fair, transparent and accountable to prevent hurting society’s most vulnerable.
Facts about fraud
First, it’s important to make one thing clear: The evidence suggests incidents of user fraud in government welfare programs are rare.
For instance, the food stamp program, formally called the Supplemental Nutrition Assistance Program, currently serves about 40 million people monthly at an annual cost of US$68 billion. Despite regular denigration of food stamp recipients, less than 1% of benefits go to ineligible households, according to the federal government.
And, of those families, the majority of overpayments result from mistakes by recipients, state workers or computer programmers as they navigate complex regulatory requirements – not any intent to defraud the system.
As for Medicaid, which provides health insurance for low-income people, research has shown that the bulk of fraudulent activity is committed by health care providers – not by the 64 million needy people that use the program.
Within unemployment insurance, the “improper payment” rate for 2019 is 10.6%, which includes payments that should not have been made or that were made in an incorrect amount, but intentional fraud estimates are much lower.
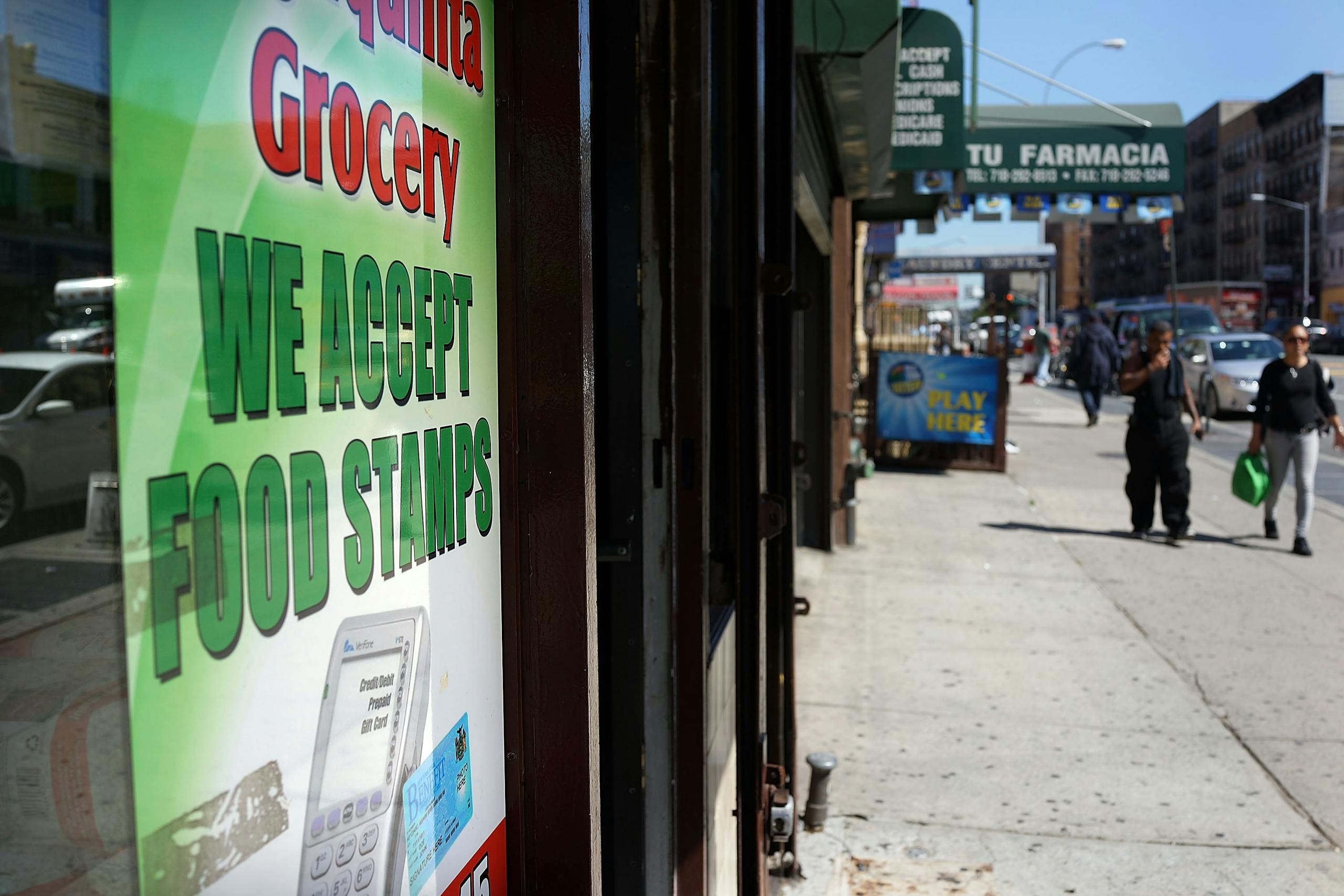
When algorithms fail
Nonetheless, many states seem to be adopting systems that assume criminal intent on the part of the needy.
Many states have begun using “sophisticated data mining” techniques to identify fraud in the food stamp program, according to the General Accountability Office. Another report identified 20 states using AI tools in unemployment insurance. And the federal government is providing support to state Medicaid programs to upgrade their decades-old technology with more advanced software.
These types of automated decision-making systems rely on algorithms, or mathematical instructions. Some algorithms use machine learning – a form of artificial intelligence – to replace decisions that would otherwise be made by humans. They analyze large sets of data to recognize patterns or make predictions.
But officials should approach these systems with caution. The results for low-income families with little margin for error can be disastrous.
For instance, in Michigan, a $47 million automated fraud detection system adopted in 2013 made roughly 48,000 fraud accusations against unemployment insurance recipients – a five-fold increase from the prior system. Without any human intervention, the state demanded repayments plus interest and civil penalties of four times the alleged amount owed.
To collect the repayments – some as high as $187,000 – the state garnished wages, levied bank accounts and intercepted tax refunds. The financial stress on the accused resulted in evictions, divorces, destroyed credit scores, homelessness, bankruptcies and even suicide.
As it turns out, a state review later determined that 93% of the fraud determinations were wrong.
How could a computer system fail so badly? The computer was programmed to detect fraud when claimants’ information conflicted with other federal, state and employer records. However, it did not distinguish between fraud and innocent mistakes, it was fed incomplete data, and the computer-generated notices were designed to make people inadvertently admit to fraud.
Michigan is not an outlier. Program-wide algorithmic errors have similarly plagued Medicaid eligibility determinations in states such as Indiana, Arkansas, Idaho and Oregon.
And the issue isn’t just an American one. Many countries such as Australia and the U.K. are embracing these types of systems and encountering similar problems. The United Nations special rapporteur on extreme poverty and human rights issued a report in October that warned governments across the world to “avoid stumbling zombie-like into a digital welfare dystopia” as they automate their social welfare systems.
In a closely watched decision, a court in the Netherlands recently halted a welfare fraud detection system, ruling that it violates human rights. The decision is likely to bring closer scrutiny to these systems worldwide, although Americans have fewer legal protections than their European counterparts.
Algorithms aren’t magic
AI won’t magically root out what little fraud there is from the welfare rolls.
Mistakes can happen when software developers translate complex regulatory requirements into code and when they make programming errors. The massive sets of data fed into automated systems inevitably will contain some inaccuracies and omissions. And algorithms can also replicate embedded societal biases and end up discriminating against marginalized groups.
Without a human in the decision-making loop, these mistakes become compounded as they flow through multiple data-sharing systems.
To avoid these problems, state and other governments should ensure the systems they install are transparent in how they function, are accountable for mistakes and don’t incentivize private contractors hired to design them to kick people off the rolls to make more money. States should also make sure representatives from all groups affected are involved in their creation and monitoring.
In my research and legal work, I have found automated fraud detection is too often built on the assumptions that computers are magic and fraud among the poor is endemic. State officials should flip those assumptions and make computers work for the people rather than against them.
[You’re too busy to read everything. We get it. That’s why we’ve got a weekly newsletter. Sign up for good Sunday reading. ]