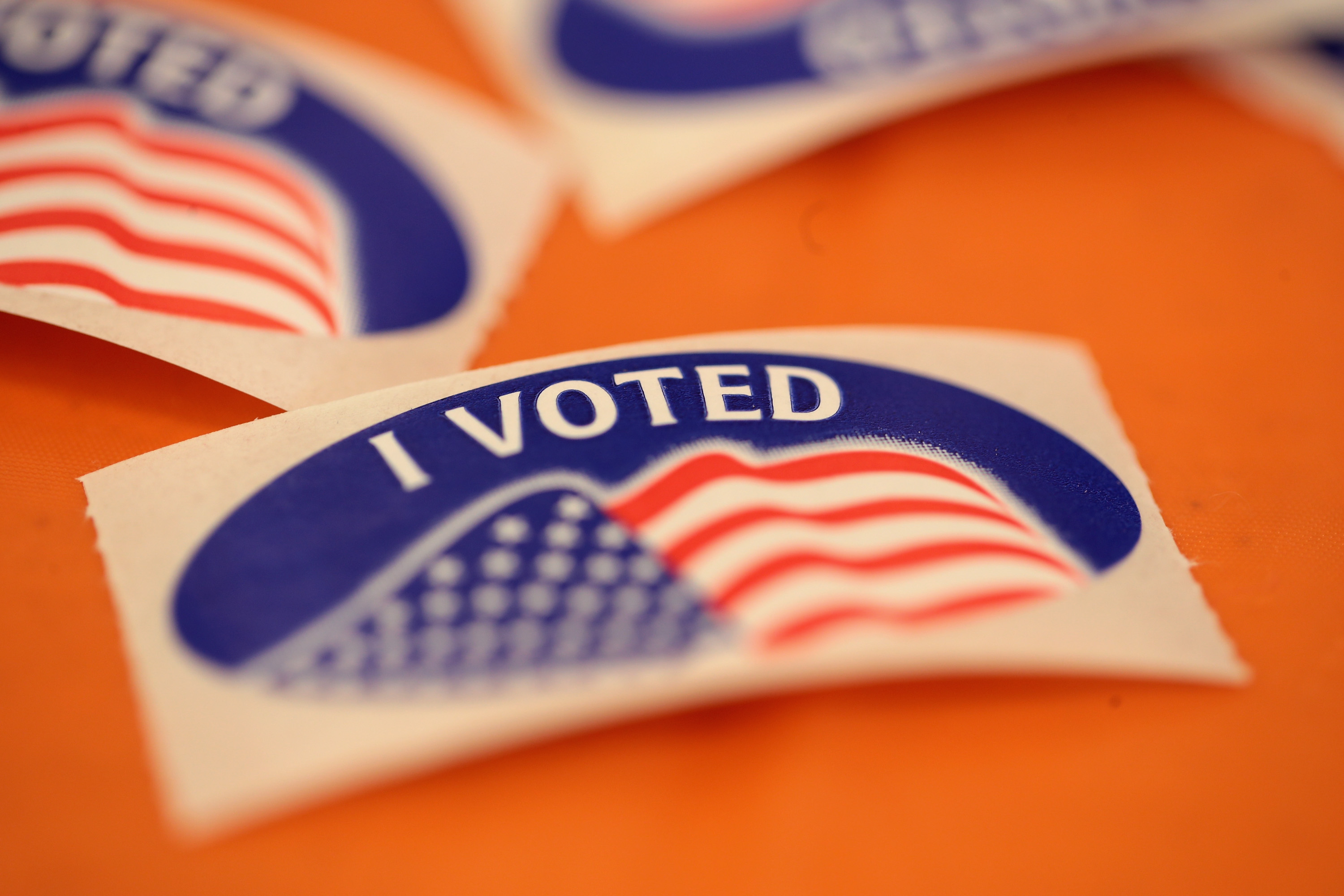
Scott Olson/Getty Images
Election polls aren’t broken, but they still can’t predict the future
Swing voters, skewed samples, and more have always limited the ability to pick a winner
by Marcus Woo, Knowable MagazineIn the days before the 2016 US presidential election, nearly every national poll put Hillary Clinton ahead of Donald Trump—up by 3%, on average. FiveThirtyEight’s predictive statistical model—based on data from state and national voter polls—gave Clinton a 71.4% chance of victory. The New York Times’ model put the odds at 85%.
Trump’s subsequent win shocked the nation. Pundits and pollsters wondered: How could the polls have been so wrong?
Trump-Clinton isn’t the only example of a recent electoral surprise. Around the world, including in the 2015 United Kingdom election, the 2015 Brexit referendum, the 2015 Israeli election, and the 2019 Australian election, results have clashed with preelection polls.
But experts contend that these misses don’t mean we should stop using or trusting polls. For example, postelection analyses of the 2016 US election suggest that national election polling was about as accurate as it has always been. (State polls, however, were a different story.) Clinton, after all, won the popular vote by 2%, not far from the 3% average that the polls found, and within the range of errors seen in previous elections. Polls failed to anticipate a Trump victory not because of any fundamental flaws, but because of unusual circumstances that magnified typically small errors.
“Everyone sort of walked away with the impression that polling was broken—that was not accurate,” says Courtney Kennedy, director of survey research at the Pew Research Center.
The issue may be one of expectations. Polls aren’t clairvoyant—especially if an election is close, which was the case in many of the recent surprises. Even with the most sophisticated polling techniques, errors are inevitable. Like any statistical measure, a poll contains nuances and uncertainties, which pundits and the public often overlook. It’s hard to gauge the sentiment of an entire nation—and harder still to predict weeks or even days ahead how people will think and act on Election Day.
“As much as I think polls are valuable in society, they’re really not built to tell you who’s going to be the winner of a close election,” Kennedy says. “They’re simply not precise enough to do that.”
Would you like to take a survey?
Pollsters do their best to be accurate, with several survey methods at their disposal. These days, polling is in the midst of a transition. While phone, mail-in and even (rarely) door-to-door surveys are still done, more and more polls are happening online. Pollsters recruit respondents with online ads offering reward points, coupons, even cash. This type of polling is relatively cheap and easy. The problem, however, is that it doesn’t sample a population in a random way. An example of what’s called a non-probability approach or convenience sampling, the Internet survey panels include only people who are online and willing to click on survey ads (or who really love coupons). And that makes it hard to collect a sample that represents the whole.
“It’s not that convenience Internet panels can’t be accurate,” says David Dutwin, executive vice president and chief methodologist of SSRS, a research survey firm that has worked on polls for outlets such as CNN and CBS News. “It’s just generally thought—and most of the research finds this—there’s certainly a higher risk with non-probability Internet panels to get inaccurate results.”
With more traditional methods, pollsters can sample from every demographic by, for instance, calling telephone numbers at random, helping ensure that their results represent the broader population. Many, if not most, of the major polls rely on live telephone interviews. With caller ID and the growing scourge of marketing robocalls, many people no longer answer calls from unknown numbers. Although response rates to phone surveys have plummeted from 36% in 1997 to 6% in 2018—a worrisome trend for pollsters—phone polls still offer the “highest quality for a given price point,” Dutwin says.
In fact, most efforts to improve the accuracy of polling set their sights on relatively small tweaks: building better likelihood models, getting a deeper understanding of the electorate (so pollsters can better account for unrepresentative samples), and coming up with new statistical techniques to improve the results of online polls.
One promising new method is a hybrid approach. For most of its domestic polls, Kennedy says, the Pew Research Center now mails invitations to participate in online polls—thus combining the ease of Internet surveys with random sampling by postal address. So far, she says, it’s working well.
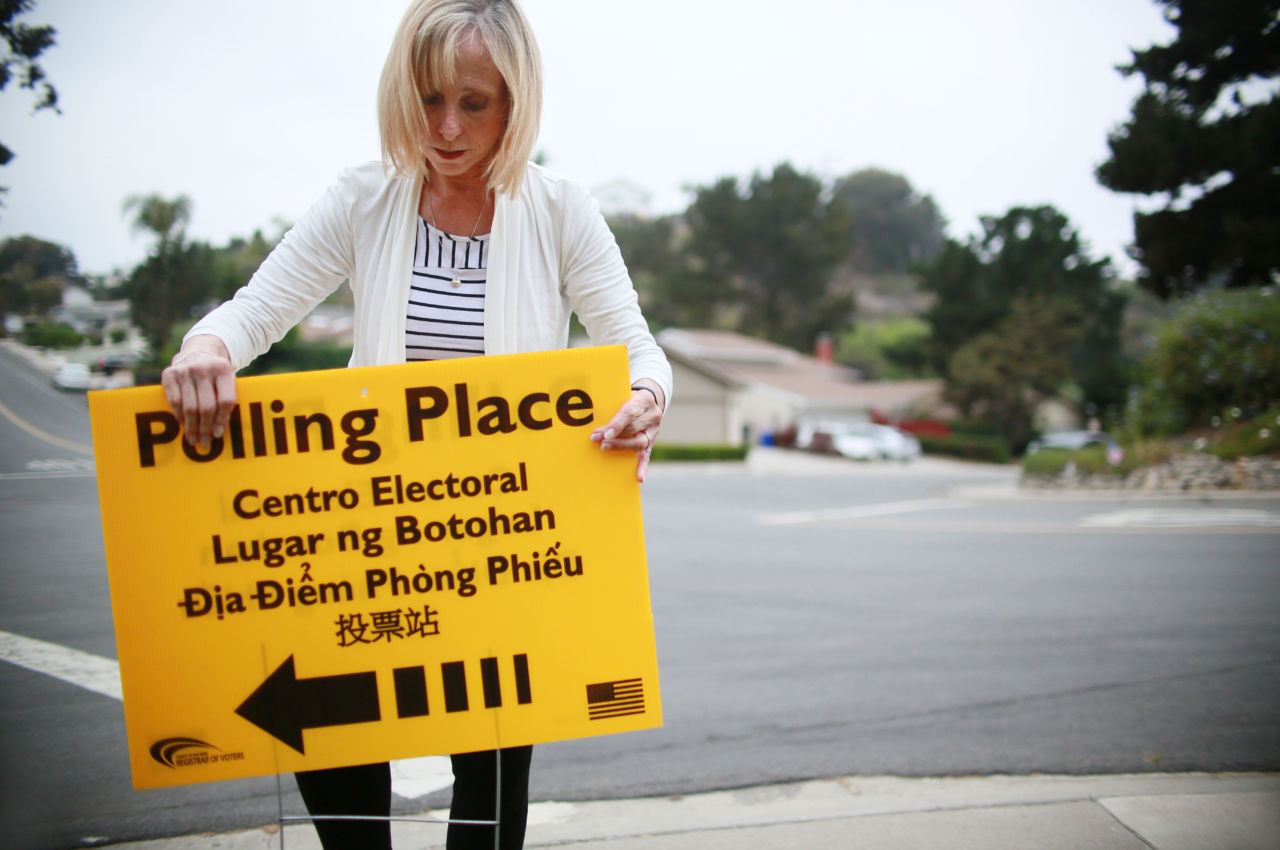
Sandy Huffaker/Getty Images
How polling goes wrong
In the spring of 2016, the American Association for Public Opinion Research formed a committee to look into the accuracy of early polling for the 2016 presidential race. After the election, the group turned to figuring out why the polls missed Trump’s victory. One primary reason, it found, was that polls—particularly state polls—failed to account for voters with only a high school education. Normally, this wouldn’t have mattered much, as voting preferences among people with different education levels tend to balance each other out. But in an election that was anything but normal, non-college-educated voters tilted toward Trump.
“College graduates are more likely to take surveys than people with less education,” says Kennedy, who chaired the AAPOR committee. “In 2016, people’s education levels were pretty correlated with how they voted for president, especially in key states.” This particular skew was unique to the 2016 election, but any kind of unrepresentative sample is the biggest source of error in polling, she says.
Pollsters have tools for predicting how skewed a sample might be and can try to correct for it by giving a proportionally larger weight to responses from any underrepresented groups. In the 2016 election, Kennedy says, many state polls didn’t perform the proper statistical weighting to account for an underrepresentation of non-college-educated voters. Some pollsters, she says, might be reluctant to apply this kind of weighting, citing the fact that they don’t know voters’ education levels ahead of time. Shoestring budgets also mean state polls tend to be of lower quality. (In 2016, their average error was 5.1%, compared to 2.2 for national polls.)
Even if pollsters can perfectly account for skewed samples, the responses to surveys could themselves be problematic. Questionnaires could be poorly designed, filled with leading questions. Or respondents might not be telling the truth. For example, voters may not want to admit their true voting preferences. This phenomenon is dubbed the Bradley Effect, named after the 1982 California gubernatorial election in which longtime Los Angeles Mayor Tom Bradley, an African American, lost to George Deukmejian, a white state official, despite leading in the polls.
According to the theory, voters would tell pollsters they would vote for the minority candidate to appear more open-minded, even though they would ultimately choose otherwise. Soon after the 2016 election, some election analysts suggested that a similar “shy Trump voter” effect may have been at play, although the AAPOR report didn’t find much evidence that it was.
Telling the future
It’s easy to write off the power of polls when they pick the wrong winner. But doing so misses the intended purpose (and acknowledged capability) of polling: to capture a snapshot of public opinion—not to make a prediction.
Most surveys ask people what they think about topics like education policy or a president's job performance at that moment in time. Election polls, on the other hand, ask people to forecast their future behavior. Asking voters whom they would pick if the election were held today—technically not about the future—poses a hypothetical situation nonetheless. This hypothetical nature of election polling is what makes it uniquely challenging.
“We’re surveying a population that doesn’t yet exist,” Dutwin says. “We don’t know who’s going to show up at the ballot box.”
To create a more accurate portrait of who’s going to show up, some pollsters have developed likely voter models, statistical models that incorporate information like a person’s voting history. They’re not perfect, but “they offer some reasonable guideposts about what the portrait is going to look like,” Kennedy says.
In the last decade, predictive models—such as the ones by the New York Times and by Nate Silver at FiveThirtyEight—have gained popularity. And these are designed to make forecasts. “One of the reasons to build a model—perhaps the most important reason—is to measure uncertainty and to account for risk,” Silver wrote three days after the 2016 election. “If polling were perfect, you wouldn’t need to do this.”
FiveThirtyEight’s most sophisticated model, for instance, incorporates polling data with demographic and economic data, tries to account for uncertainty, and then simulates thousands of elections to produce a probability of victory. These models also take the electoral college into account, which election polls don’t (probably another reason polls failed to pick Trump).
Still, these models depend on accurate polls, and no matter your statistical tool, you can never truly predict the future. Sometimes, voters change their minds. Or they don’t show on Election Day. And some voters don’t decide until the last minute. In fact, in addition to the undercounting of non-college-educated voters, this kind of late swing was the other major reason polls missed in 2016, according to the AAPOR report. Undecided voters in battleground states swung strongly in Trump’s direction—by as much as 29% points in Wisconsin, for example. In most elections, Kennedy says, late swings end in a wash, with undecided voters going equally in both directions.
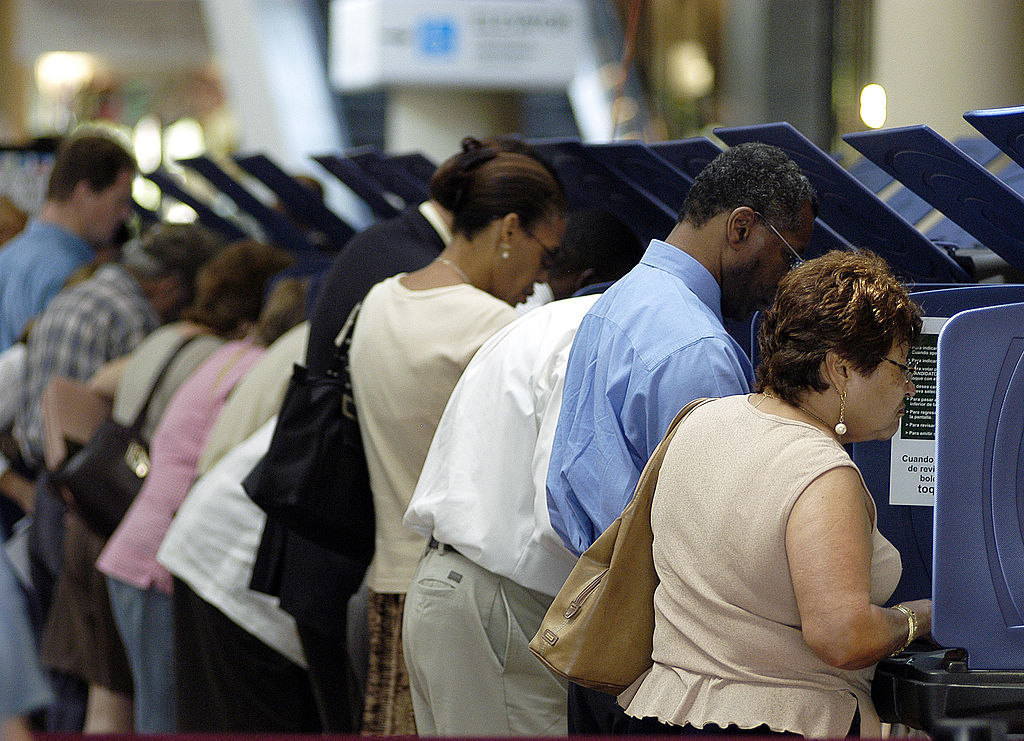
Polling for democracy
Once the shock wears off, discrepancies between polls and actual results usually aren’t as wide as they initially seem. In 2016, people were too complacent about Clinton’s chances—despite actual poll data and their uncertainties, according to Silver. Just looking at the numbers, he wrote, “We think people should have been better prepared for it.”
Pollsters and journalists thus need to be better at communicating uncertainties, says Ron Kenett, an applied statistician at the Samuel Neaman Institute at the Technion in Haifa, Israel, who recently wrote about polling issues in the Annual Review of Statistics and Its Application. The public doesn’t usually consider statistical uncertainties, he says. “People will get hung up on supposedly clear pictures even though they are blurred.”
While election polls will never provide a crystal clear view of the future, they’re still necessary for taking the pulse of the electorate. “Polls are a critical tool for democracy,” Dutwin says. “Democracy requires that we know what we think about things, because otherwise, the politicians are just going to tell us what we think.”
Marcus Woo is a freelance science journalist based in the San Francisco Bay Area. Follow him @sucramoow.
This article originally appeared in Knowable Magazine, an independent journalistic endeavor from Annual Reviews. Sign up for the newsletter.